Free webinar
How relevant is data modeling in modern big data platforms?
Traditional data modeling techniques like Kimball or Data Vault give way to modern machine learning use cases. Moreover they fail to rise in volume and variety of unstructured data.
Modern, hybrid architectures such as Data Lakehouse try to tackle it by creating a unified data management layer, But is this enough to untangle the data spaghetti?
Learn more:
How to overcome data modeling challenges in modern data platforms
Why you need a new form of data modeling for unstructured data and ML use cases
How to avoid data chaos
Modern data platforms require modern data modelling techniques
Most data platforms need traditional data warehouses to cover daily reports and BI. But the use of unstructured data in machine learning requires an evolution of data modelling techniques. In this webinar we’ll talk about it in detail. Make sure to stick around for the Q&A at the end of the webinar.
Is Data Lakehouse enough?
Modern solutions like Data Lakehouse offer a unified Metadata and Data Governance Layer. Let’s take a peek together on how to decide on the best shape(s) of your data.
Schema-on-read vs schema-on-write, is there more?
Unstructured data requires a dynamically created model. However, structured data doesn’t, but has its limitations. In some cases you need a hybrid solution to overcome challenges behind creating operational ML solutions and real-time reporting.
Speakers
Mikołaj Kromka
Team Lead and Principal Software Engineer at VirtusLab
His background covers 10 years in the IT business with knowledge of Java, Scala, big data and machine learning engineering. Currently, he implements stable and reliable software solutions in data platforms for clients worldwide.
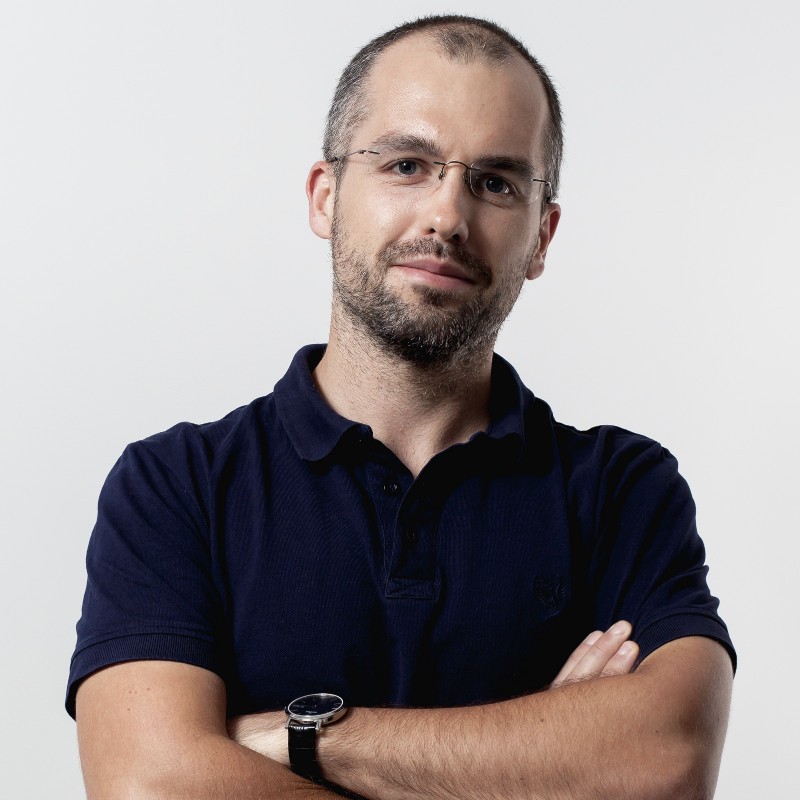
Wojciech Nowak
Senior Data Engineer at VirtusLab
Software Engineer with 6 years of professional experience, focused on delivering end-to-end, robust and reliable solutions in big data and software engineering.
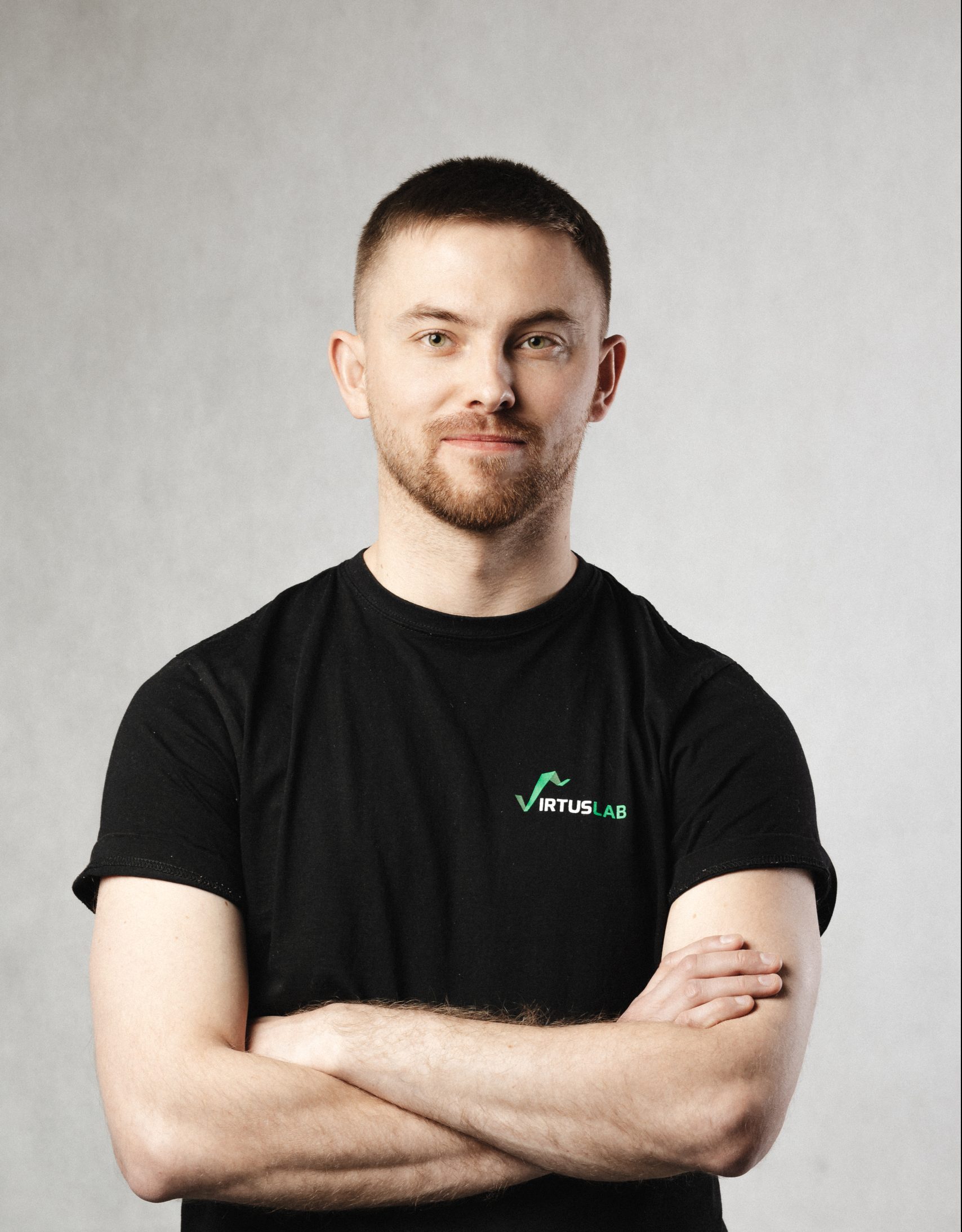